Author: Jeff Pawelek –
Throughout the COVID-19 pandemic, public health agencies fulfilled a crucial role in helping communities track and mitigate the spread of SARS-CoV-2. In the pandemic’s early stages, an active reporting system was developed using case surveillance data from hospitals, healthcare providers, and clinical laboratories. The case surveillance data is pooled and analyzed by public health officials on an ongoing basis to notify communities about local outbreaks as they happen in real-time and to inform public health safety measures.
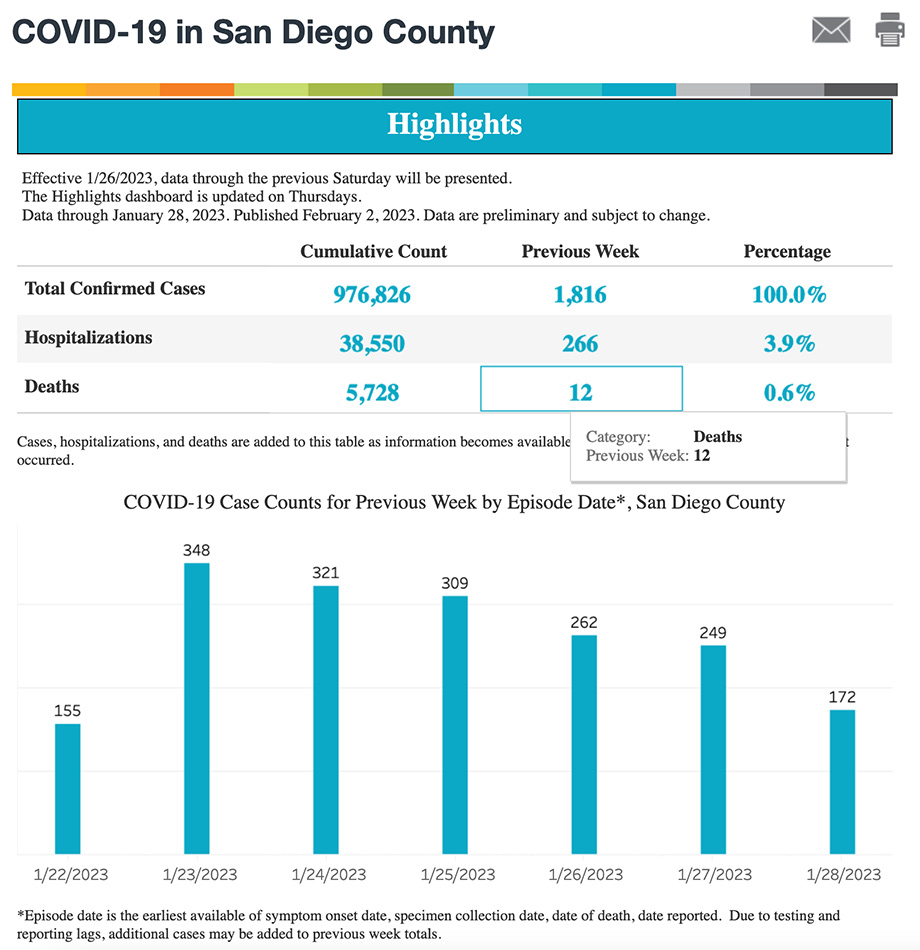
Credit: San Diego County’s COVID-19 dashboard (screenshot taken February 6, 2023). Source: https://www.sandiegocounty.gov/content/sdc/hhsa/programs/phs/community_epidemiology/dc/2019-nCoV/status.html
Recently, at-home rapid antigen testing gained in popularity due to it being widely available, convenient, and affordable (or free). However, most people do not share their at-home test results with their healthcare provider or local health agency, thus a significant amount of testing data is no longer accounted for by traditional public health counts.
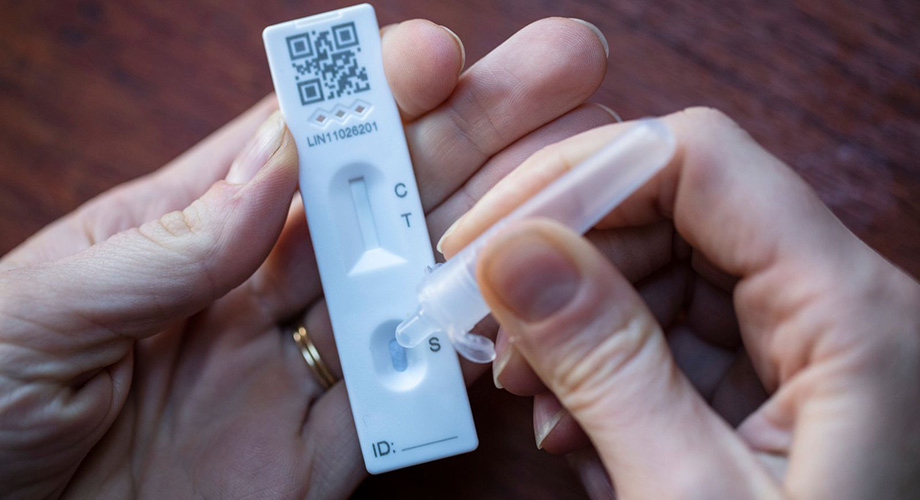
In an effort to fill this information gap, public health officials have looked to passive monitoring strategies, such as wastewater monitoring, to improve the accuracy in calculating the incidence of new COVID-19 cases. Additionally, the increasing use of mobile health technologies (e.g. wearables, smartphones/watches, health apps) among consumers has created novel passive strategies for detecting and tracking viral infections. Several published reports, including our own Digital Engagement and Tracking for Early Control and Treatment (DETECT) study, have shown the utility of wearables to detect COVID-19 infection using heart rate, sleep and physical activity information including app-based reporting of symptoms and at-home rapid test results. [1, 2, 3, 4]
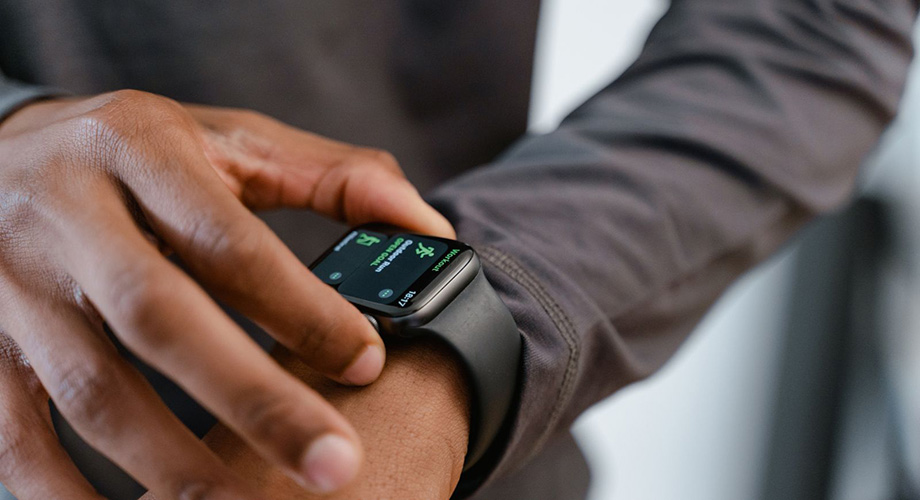
In a recent article published by the Scripps Research Digital Trials Center in collaboration with the Robert Koch Institute – a German federal government agency and research institute responsible for disease control and prevention – the research team compared traditional case surveillance data to self-reported at-home test results that were collected through two large scale app-based studies. Data from the app-based studies included the US-based DETECT study and the Germany-based Corona-Datenspende (CDA) study, equating to a total of over 200,000 at-home test results. The goal was to determine if there were differences in the number of new COVID-19 cases between traditional surveillance methods and app-based case reporting strategies as testing started to shift from taking place in a clinic or laboratory to the home. International collaboration allowed for replication of the findings in two different countries further supporting the potential benefit of digital surveillance tools to inform public health.
The comparison found that self-reported positive test numbers correlated well with traditional surveillance numbers. It also found that self-reported test numbers started to track higher than traditional surveillance numbers around the time when home antigen tests became more widely available. These results demonstrate the feasibility of using app-based reporting to complement traditional surveillance methods, potentially improving the accuracy of official public health case counts. By combining both active and passive reporting strategies, public health officials can be better positioned to issue effective safety measures to help communities curb the spread of COVID-19.
The study – ‘Prevalence of Positive COVID-19 Test Results Collected by Digital Self-report in the US and Germany’ – is available on JAMA Network Open.
References:
- Radin JM, Quer G, Ramos E, Baca-Motes K, Gadaleta M, Topol EJ, Steinhubl SR. Assessment of Prolonged Physiological and Behavioral Changes Associated With COVID-19 Infection. JAMA Netw Open. 2021 Jul 1;4(7):e2115959. doi: 10.1001/jamanetworkopen.2021.15959. PMID: 34232306; PMCID: PMC8264646.
- Gadaleta M, Radin JM, Baca-Motes K, Ramos E, Kheterpal V, Topol EJ, Steinhubl SR, Quer G. Passive detection of COVID-19 with wearable sensors and explainable machine learning algorithms. NPJ Digit Med. 2021 Dec 8;4(1):166. doi: 10.1038/s41746-021-00533-1. PMID: 34880366; PMCID: PMC8655005.
- Radin JM, Quer G, Pandit JA, Gadaleta M, Baca-Motes K, Ramos E, Coughlin E, Quartuccio K, Kheterpal V, Wolansky LM, Steinhubl SR, Topol EJ. Sensor-based surveillance for digitising real-time COVID-19 tracking in the USA (DETECT): a multivariable, population-based, modelling study. Lancet Digit Health. 2022 Nov;4(11):e777-e786. doi: 10.1016/S2589-7500(22)00156-X. Epub 2022 Sep 22. PMID: 36154810; PMCID: PMC9499390.
- Quer G, Gadaleta M, Radin JM, Andersen KG, Baca-Motes K, Ramos E, Topol EJ, Steinhubl SR. Inter-individual variation in objective measure of reactogenicity following COVID-19 vaccination via smartwatches and fitness bands. NPJ Digit Med. 2022 Apr 19;5(1):49. doi: 10.1038/s41746-022-00591-z. PMID: 35440684; PMCID: PMC9019018.