Authors: Lauren Serpico & Jeff Pawelek –
February is recognized as American Heart Month and for good reason – heart disease remains the leading cause of death among adults in the United States. There is a lot we can do to protect our heart, and wearable activity trackers and smartwatches have become valuable tools in the quest towards optimal heart health by tracking key metrics such as heart rate, activity level, and sleep duration.
One of the most common heart conditions is atrial fibrillation or AF [1], which is when the heart beats irregularly and faster than normal. AF can lead to heart failure or stroke, and early detection and management can help patients avoid serious complications. However, 10-20% of people who have AF are not diagnosed, even after a traditional 2-week cardiac monitoring period [2, 3].
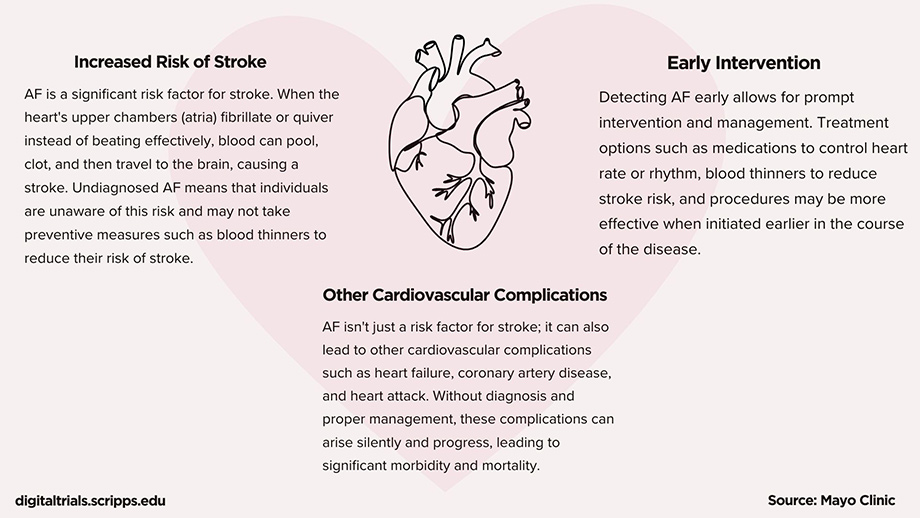
Recent findings from a Scripps Research digital study
In a recent study led by the Scripps Research Translational Institute, and in collaboration with iRhythm, artificial intelligence (AI) was used to develop a new AF detection method [4].
The study used a novel wearable device (in the form of a small chest patch) (Figure 1). The wearable patch recorded the heart’s electrical activity (known as an electrocardiogram or ECG), and deep learning techniques developed by Scripps Research scientists were used to analyze ECG recordings from over 446,000 study participants, including patient demographics and other heart rhythm parameters.
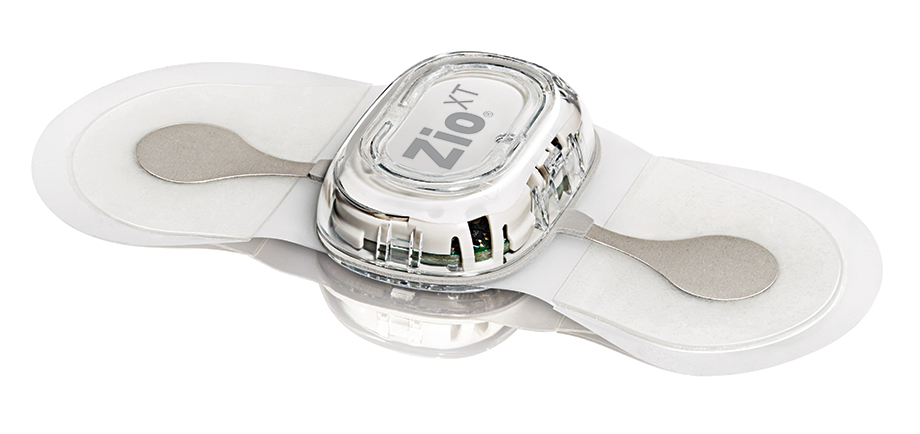
Figure 1. iRhythm Zio® XT patch, an FDA cleared continuous ambulatory single-lead ECG skin adhesive patch (iRhythm Technologies Inc, San Francisco, CA).
The AI-powered analysis produced an accurate model for the early detection of AF. The study findings demonstrate how wearable devices and AI techniques can aid in the early detection of serious heart conditions, which signals a significant step forward in using novel technologies to improve diagnostic capabilities and early treatment.
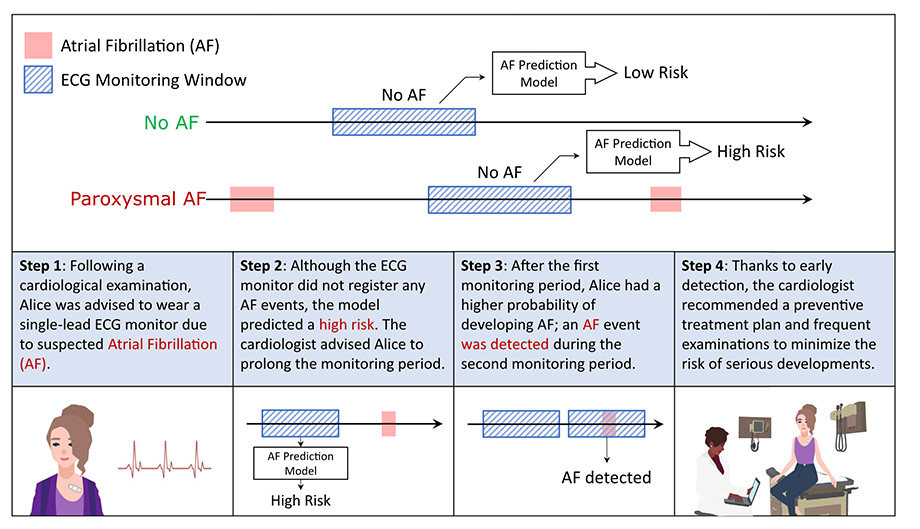
Figure 2. An example of application of the AF prediction model. The figure illustrates the possible outcomes of the AF prediction model when AF is not detected: it can predict low or high risk for future AF development. In the second part of the figure, we present an example of a potential application. Alice was advised to wear an ECG patch to monitor potential AF, and although no AF was detected, the model predicted a high risk of AF. Consequently, she was advised to wear a second ECG patch, which ultimately detected AF, allowing her to discuss appropriate treatment with her clinician. Source: npj Digital Medicine
Wearables for early detection of heart conditions in the real world
We live our lives outside the clinic, but until recently, cardiological diagnostic testing such as ECG took place primarily within the confines of a medical office or hospital. As a result, physicians and scientists had access to just a trickle of data, which painted an incomplete — or even inaccurate — picture.
Heart health has been an area of research that we have been exploring since 2015. Our mSToPS (mHealth Screening To Prevent Strokes) trial equipped participants with an AF-detecting ECG-sensor patch that could be worn 24 hours a day up to 14 days. It was the first AF study to be conducted entirely at home, without any need to visit a medical facility. After a year of follow-up, the research team determined that mSToPS participants were three times more likely to be accurately diagnosed with AF, compared with those who had received usual care.
In the summer of 2018, we published this pioneering digital clinical trial in the Journal of the American Medical Association. It was a groundbreaking, nationwide, site-less, digital medicine study, showcasing the power of implementing digital technologies in clinical research.
The following are publications derived from this digital clinical trial:
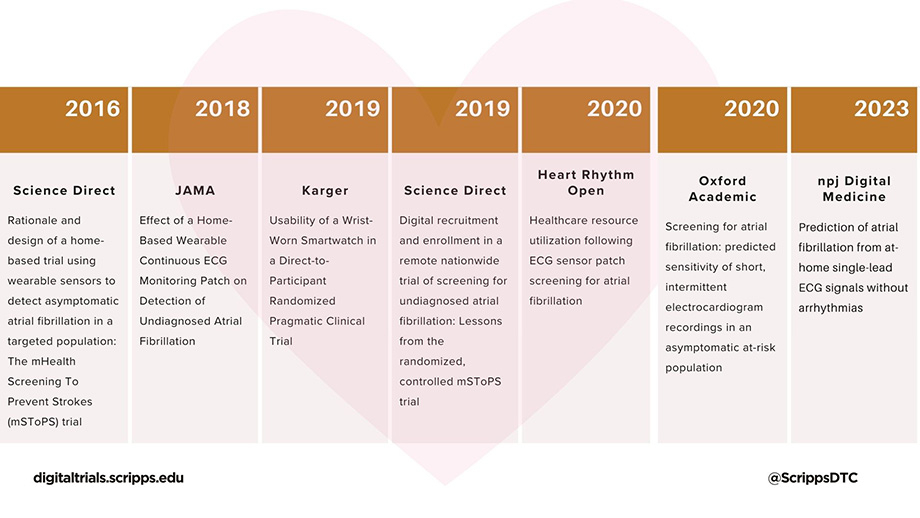
To explore each article listed, visit:
- Prediction of atrial fibrillation from at-home single-lead ECG signals without arrhythmias (2023)
- Screening for atrial fibrillation: predicted sensitivity of short, intermittent electrocardiogram recordings in an asymptomatic at-risk population (2020)
- Healthcare resource utilization following ECG sensor patch screening for atrial fibrillation (2020)
- Digital recruitment and enrollment in a remote nationwide trial of screening for undiagnosed atrial fibrillation: Lessons from the randomized, controlled mSToPS trial (2019)
- Usability of a Wrist-Worn Smartwatch in a Direct-to-Participant Randomized Pragmatic Clinical Trial (2019)
- Effect of a Home-Based Wearable Continuous ECG Monitoring Patch on Detection of Undiagnosed Atrial Fibrillation (2018)
- Rationale and design of a home-based trial using wearable sensors to detect asymptomatic atrial fibrillation in a targeted population: The mHealth Screening To Prevent Strokes (mSToPS) trial (2016)
Digital innovation towards a heart-healthy future
As we navigate through American Heart Month, we highlight the advances in technology that provide a tangible reality in reshaping how we approach heart health. From wearable trackers to advancements in AI-analysis showcased in the literature, the landscape of cardiovascular care is undergoing a paradigm shift. Digital clinical trials such as mSToPS show the potential to revolutionize patient outcomes. By embracing this innovation we are not only redefining the boundaries of clinical research, we are empowering individuals to take proactive steps towards their health.
References
- Go AS, Hylek EM, Phillips KA, Chang Y, Henault LE, Selby JV, Singer DE. Prevalence of diagnosed atrial fibrillation in adults: national implications for rhythm management and stroke prevention: the AnTicoagulation and Risk Factors in Atrial Fibrillation (ATRIA) Study. JAMA. 2001 May 9;285(18):2370-5. doi: 10.1001/jama.285.18.2370. PMID: 11343485.
- Turakhia MP, Guo JD, Keshishian A, Delinger R, Sun X, Ferri M, Russ C, Cato M, Yuce H, Hlavacek P. Contemporary prevalence estimates of undiagnosed and diagnosed atrial fibrillation in the United States. Clin Cardiol. 2023 May;46(5):484-493. doi: 10.1002/clc.23983. Epub 2023 Mar 1. PMID: 36855960; PMCID: PMC10189075.
- Turakhia MP, Shafrin J, Bognar K, Trocio J, Abdulsattar Y, Wiederkehr D, Goldman DP. Estimated prevalence of undiagnosed atrial fibrillation in the United States. PLoS One. 2018 Apr 12;13(4):e0195088. doi: 10.1371/journal.pone.0195088. PMID: 29649277; PMCID: PMC5896911.
- Gadaleta M, Harrington P, Barnhill E, Hytopoulos E, Turakhia MP, Steinhubl SR, Quer G. Prediction of atrial fibrillation from at-home single-lead ECG signals without arrhythmias. NPJ Digit Med. 2023 Dec 12;6(1):229. doi: 10.1038/s41746-023-00966-w. PMID: 38087028; PMCID: PMC10716265.