Authors: Mattia Carletti, Jeff Pawelek & Giorgio Quer –
Annotations of patients’ health status have been recorded on paper and organized in folders for centuries and have played a key role in how providers make clinical decisions and deliver care. The introduction of computers paved the way for a revolution in how we collect, manage and use health data through the development of electronic health record (EHR) systems. The diffusion of EHR, combined with advancements in digital health technologies, has led to an explosion of health data and opened up endless opportunities to integrate these data as a way to modernize patient care and health research.
Artificial intelligence and the power to predict health outcomes
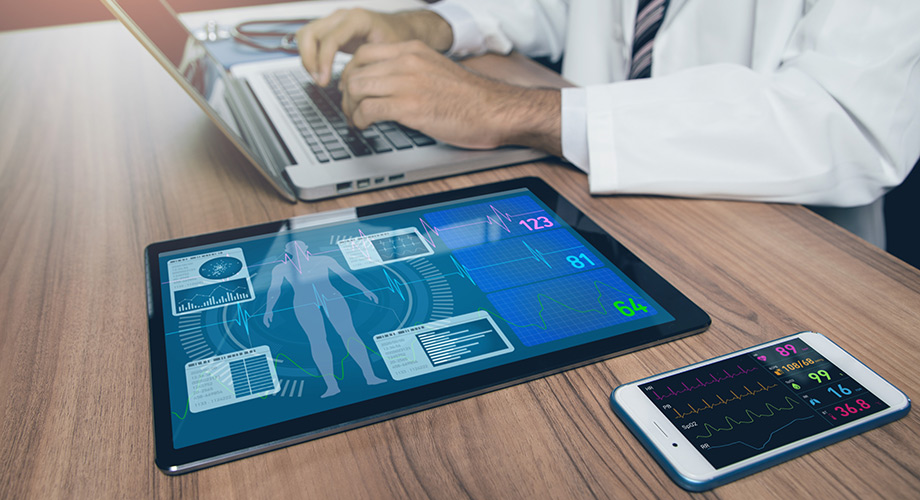
The availability of massive and detailed health datasets enabled the use of Artificial Intelligence (AI) to solve challenging clinical problems that would have been unthinkable only a decade ago. AI is broadly defined as the discipline devoted to the development of technologies to empower machines with capabilities that are typical of human intelligence. Machine Learning (ML) is arguably the most famous subfield of AI, which is used to create algorithms that are able to extract patterns and rules to make predictions without being explicitly programmed to do so. Scientists at the Scripps Research Digital Trials Center are leading pioneering studies in this field.
For example, we developed ML models to discriminate between individuals who tested positive or negative for COVID-19 based on metrics derived from wrist-worn wearable device data, demographic information and self-reported symptoms [1, 2]. These ML models enable the early identification of symptomatic and pre-symptomatic infected individuals, which could be extremely helpful to improve contact tracing and limit the spread of disease. Additionally, in another study, smartwatch data, combined with self-reported symptoms, COVID-19 test results and vaccine information, have been used to derive digital biomarkers (i.e., measures derived from digital devices data that are useful to predict health outcomes) for how individuals respond to COVID vaccines [3]. The potential to objectively measure the immune response to vaccines not only provides a scalable and non-invasive alternative to blood tests, but could also inform future personalized vaccine policies.
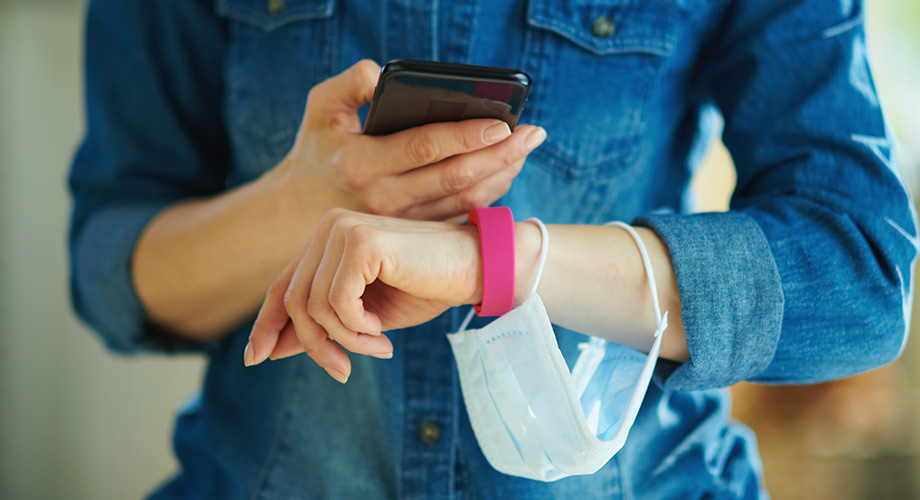
In an ongoing study led by the Scripps Research Digital Trials Center, we tackle challenging problems in the field of precision nutrition through the collection of multimodal data from fitness trackers, continuous glucose monitors, food tracking apps, EHR, and self-collected stool, blood and saliva samples. The objective is to design ML models that could improve glycemic control (e.g., by predicting an individual’s glycemic response to a particular food) or help prevent Type 2 Diabetes by estimating the risk of developing the disease for healthy individuals.
The use of ML proved to be successful also in the medical image domain. For example, in a study led by University College London (UCL) and DeepMind, a ML model designed to automatically diagnose retinal diseases and provide referral suggestions based on 3-dimensional optical coherence tomography scans showed performance on par or better when compared with human experts [4]. This work demonstrates how ML models can equip clinicians with advanced clinical support tools to deliver care more efficiently and accurately. Pearse Keane, Professor of Artificial Medical Intelligence at UCL, one of the leading authors of the study, visited Scripps Research last month to explain his research.
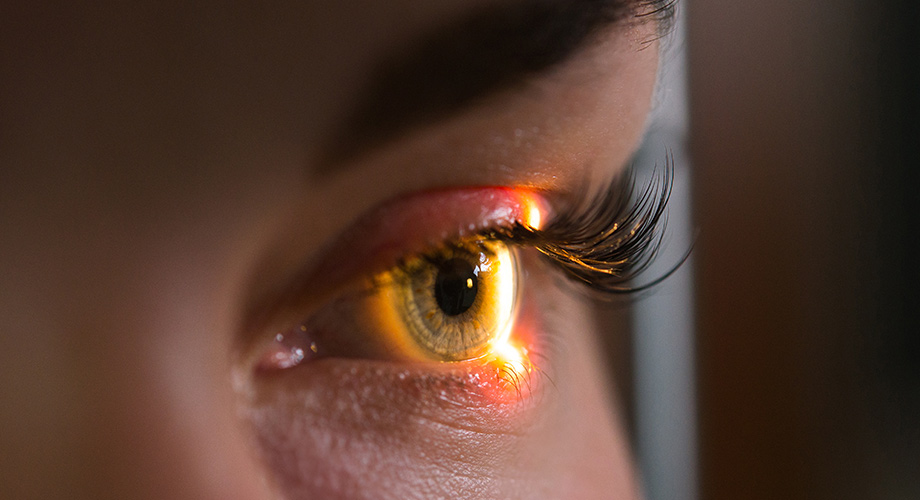
The future of AI in healthcare
AI has already achieved impressive results in supporting medical professionals and advancing knowledge discovery in medicine. Still, its full potential has yet to be unlocked. Initial applications of AI in healthcare have primarily focused on medical imaging analysis, yet recent advances in AI – such as large language models like ChatGPT – will surely boost performance of existing algorithms and push the limits beyond what we ever thought possible. Particularly for ChatGPT, there are practical considerations to sort out before any patient-facing application in healthcare, since these models can potentially show unexpected behavior (referred to as “hallucinations”) or report misinformation, thus requiring a careful human review to protect patient safety and privacy. One thing is certain: AI technologies will shape the future of data-driven medical research and patient care, and healthcare is poised to become one of the most emblematic examples of AI at the service of humanity.
References:
- G. Quer, J. M. Radin, M. Gadaleta, K. Baca-Motes, L. Ariniello, E. Ramos, V. Kheterpal, E. J. Topol and S. R. Steinhubl, “Wearable sensor data and self-reported symptoms for COVID-19 detection,” Nature Medicine, vol. 27, p. 73–77, 2021.
- M. Gadaleta, J. M. Radin, K. Baca-Motes, E. Ramos, V. Kheterpal, E. J. Topol, S. R. Steinhubl and G. Quer, “Passive detection of COVID-19 with wearable sensors and explainable machine learning algorithms,” NPJ Digital Medicine, vol. 4, p. 166, 2021
- G. Quer, M. Gadaleta, J. M. Radin, K. G. Andersen, K. Baca-Motes, E. Ramos, E. J. Topol and S. R. Steinhubl, “Inter-individual variation in objective measure of reactogenicity following COVID-19 vaccination via smartwatches and fitness bands,” npj Digital Medicine, vol. 5, p. 49, 2022
- De Fauw J, Ledsam JR, Romera-Paredes B, Nikolov S, Tomasev N, Blackwell S, Askham H, Glorot X, O’Donoghue B, Visentin D, van den Driessche G, Lakshminarayanan B, Meyer C, Mackinder F, Bouton S, Ayoub K, Chopra R, King D, Karthikesalingam A, Hughes CO, Raine R, Hughes J, Sim DA, Egan C, Tufail A, Montgomery H, Hassabis D, Rees G, Back T, Khaw PT, Suleyman M, Cornebise J, Keane PA, Ronneberger O. Clinically applicable deep learning for diagnosis and referral in retinal disease. Nat Med. 2018 Sep;24(9):1342-1350.